point cloud processing -凯发k8网页登录
a point cloud is a set of data points in 3-d space. the points together represent a 3-d shape or object. each point in the data set is represented by an x, y, and z geometric coordinate. point clouds provide a means of assembling a large number of single spatial measurements into a dataset that can be represented as a describable object. point cloud processing is used in robot navigation and perception, depth estimation, stereo vision, visual registration, and in advanced driver assistance systems (adas). computer vision toolbox™ algorithms provide point cloud processing functionality for downsampling, denoising, and transforming point clouds. the toolbox also provides point cloud registration, geometrical shape fitting to 3-d point clouds, and the ability to read, write, store, display, and compare point clouds. you can also combine multiple point clouds to reconstruct a 3-d scene.
you can use , , , and to register a moving point cloud to a fixed point cloud. these registration algorithms are based on the iterative closest point (icp) algorithm, the normal-distributions transform (ndt) algorithm, the phase correlation algorithm, and the coherent point drift (cpd) algorithm, respectively. you can build a map with the registered point clouds, detect loop closures, optimize the map to correct for drift, and perform localization in the prebuilt map. for more details, see .
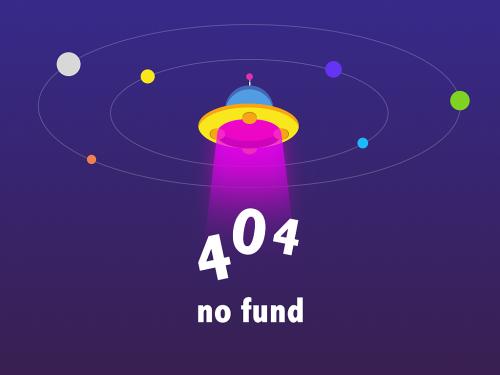
functions
blocks
topics
compare visualization functions.
- choose slam workflow based on sensor data
choose the right simultaneous localization and mapping (slam) workflow and find topics, examples, and supported features.
understand point cloud registration and mapping workflow.
the stanford triangle format
- getting started with point clouds using deep learning
understand how to use point clouds for deep learning.
compare visualization functions.
- labeling, segmentation, and detection (lidar toolbox)
label, segment, detect, and track objects in point cloud data using deep learning and geometric algorithms